Tauhidur Rahman, Ph.D.
Assistant Professor
Halıcıoğlu Data Science Institute
University of California San Diego
https://mosaic-laboratory.
Seminar Information
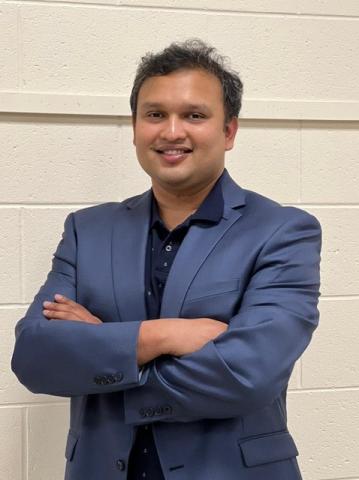
Personal and population health applications built on top of large-scale mobile sensor data and computing platforms have a great potential to impact the way we diagnose diseases, track, and manage our health. However, the existing sensing mechanisms often fail to accurately capture and infer syndromic signatures that are indicative of anomalies in internal physiological and behavioral processes at an earlier stage. A mobile sensing system that can harness early syndromic signals at an individual or a community level can pave the way to effective just-in-moment intervention, early screening, and prevention.
In this talk, I will present our recent and ongoing research to demonstrate how physiological time series data harnessed from on-body wearable systems can be used for modeling opioid use/administration, affective states including craving, pain, stress and euphoria, and opioid misuse. I will talk about different approaches (attention based approach and Large Language Model based approach) to fuse multimodal physiological biomarker data, behavioral data, clinical health record data, demographic data as well as symptom data. I will highlight how integration of pharmacological knowledge such as Pharmacokinetics of a specific substance can help neural networks to better generalize and learn opioid related physiological events better than a purely data driven approach.
Chronic opioid use induces neuroplastic changes in brain circuits, causing predictable changes in different states including heightened stress, increased pain, and intense cravings. The fluctuations or changes in stress, pain and craving states carries telltale signal for opioid misuse risk. In the last part of the talk, I will present how the heart rate variability data from a wearable wristband can be used to predict momentary pain, stress and craving state trajectories with a personalized hierarchical deep learning model, obviating the need for obtrusive ecological momentary assessments throughout the day. We adopt a nonlinear dynamical systems approach with different features including persistence entropy to extract subtle trends from the moment-by-moment fluctuations or changes in pain, stress and craving states. Our analysis reveals a hidden counter-intuitive association between high entropy or lack of predictability (i.e., chaos) in the momentary pain, stress and craving states with the decrease in opioid misuse risk. Leveraging Chaos Theory, the entropy-based nonlinear dynamical features can be used to train a deep learning based approach for accurate opioid misuse risk assessment.
Tauhidur Rahman is an Assistant Professor in the Halıcıoğlu Data Science Institute and Computer Science and Engineering at the University of California San Diego where he directs the Mobile Sensing and Ubiquitous Computing Laboratory (MOSAIC Lab). His current research focuses on building novel ubiquitous and mobile health sensing technologies that capture observable low-level physical signals in the form of an acoustic and electromagnetic wave from our bodies and surrounding environments and map them to relevant biological and behavioral measurements. Some of his notable accomplishments include a Google Research Scholar Award 2023, a Google Ph.D. fellowship in 2016 in mobile computing, a finalist position in Qualcomm innovation fellowship in 2015, Outstanding Teaching Award 2015 from Cornell University, one best paper award in ACM Digital Health 2016, one best paper honorable mention award in ACM Ubicomp 2015 and a distinguished paper award from ACM IMWUT in 2021. Tauhidur received his B.S. in Electrical and Electronic Engineering from the Bangladesh University of Engineering and Technology, his M.S. in Electrical Engineering from the University of Texas at Dallas and PhD in Information Science from Cornell University. He has a long track-record working with large-scale multi-modal and multi-rate sensor data, especially in the application areas of digital epidemiology, substance use disorder, mental health and sleep. His work has been featured in several US-based and International media outlets including Wall Street Journal, MIT Technology Review, NewScientist, Public Television for Western New England, Daily Mail (UK) and Hindustan Times (India). His laboratory has been funded by NSF, NIH, DARPA and industry grants.